Using machine learning to identify incentives in forestry policy: Towards a new paradigm in policy analysis
November 2021
Forest Policy and Economics 134
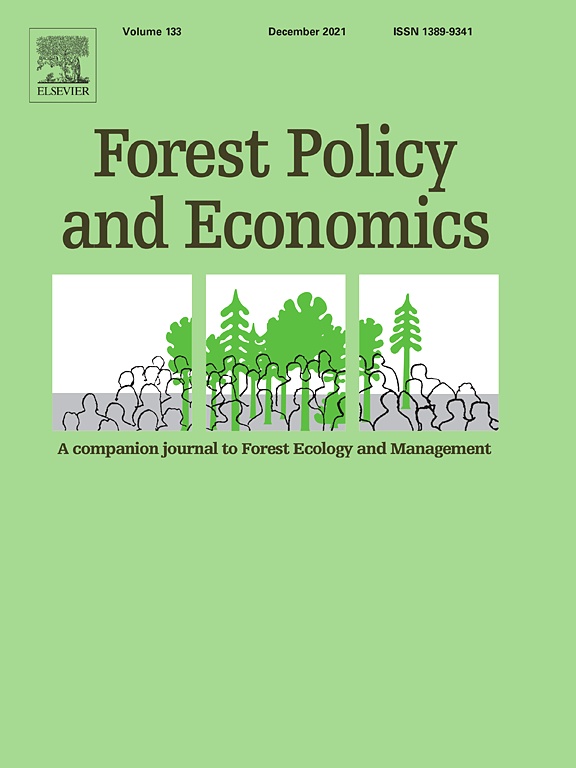
As 2021 saw the launch of the United Nations Decade on Ecosystem Restoration, it highlighted the need to prepare for success over the decade and to understand what public economic and financial incentives exist to support sustainable forest and landscape restoration. To date, Initiative 20x20 has committed to placing 50 million hectares under restoration and conservation by 2030. Understanding the public policies in these countries that turn those commitments into action, however, is very labor intensive, requiring decision makers to read and analyze thousands of pages of documents that span multiple sectors, ministries, and scales that lie outside of their areas of expertise. To address this, we developed a semiautomated policy analysis tool that uses state-of-the-art Natural Language Processing (NLP) methods to mine policy documents, assist the labeling process carried out by policy experts, automatically identify policies that contain incentives and classify them by incentive instrument from the following categories: direct payments, fines, credit, tax deduction, technical assistance and supplies. Our best model achieves an F1 score of 93–94% in both identifying an incentive and its policy instrument, as well as an accuracy of above 90% for 5 out of 6 policy instruments, reducing multiple weeks of policy analysis work to a matter of minutes. In particular, the model properly identified the relative frequency of credits, direct payments, and fines that exist as the primary policy instruments in these countries. We also found that tax deductions, supplies, and technical assistance are much less used among most of the countries and that, oftentimes, the policy documents describe economic incentives for restoration in vague and intangible terms. In addition, our model is designed to constantly improve its performance with more data and feedback from policy experts. Furthermore, while our experiments were run on Spanish policy documents, we designed our framework to be widely scalable to policies from different countries and multiple languages, limited only by the number of languages supported by current multilingual NLP models. Using a standardized approach to generate incentives data could provide an evidence-based and transparent system to find complementarity between policies and help remove barriers for implementers and policymakers and enable a more informed decision-making process.